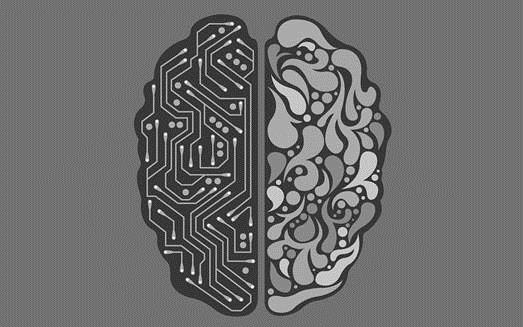
Over the last few years, artificial intelligence (AI) has been the subject of a ream of media reports and conferences, including reports of how many jobs will now be obsolete. However much AI may promise, in reality very few companies hold enough data to train AI and even for those that do, the prohibitive costs of labeling data and the current supervised learning method leaves them not much better off.
Alex Hou, of the Industrial Economics and Knowledge Center of the Industrial Technology Research Institute (ITRI), stated at a recent conference in Taipei, that although these barriers are still currently in place, there are some companies actively working to resolve them. Once these issues are resolved, this may trigger more uptake of AI technologies among small and medium-sized firms.
Hou stated that there have been two approaches to overcoming this problem; the first being to lower the threshold for the quantity of data required and the second being the creation of data through generative adversarial networks and reinforcement learning.
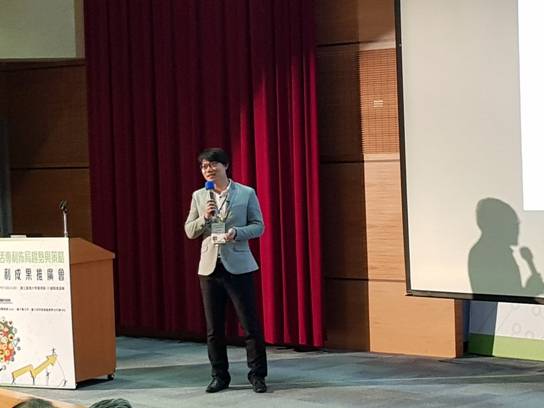 Alex Hou speaking at the conference in Taipei; Source: Conor Stuart
The first of these involves forming algorithms which mimic the human brain. Numenta and Geometric Intelligence are two of the companies that are attempting to reverse engineer the neo-cortex. Geometric Intelligence was famously acquired by Uber towards the end of 2016.
Numenta states its mission as “researching how the neocortex works with the initial purpose of understanding, and a secondary aim of developing technology on this basis.” By understanding how humans can carry out tasks with a small amount of data, it is hoped that machines can learn in a similar way, so less data is required. Hou pointed to the way humans learn to drive vs the amount of training needed for a machine to drive a car as an example. While humans can learn in dozens of hours, AI requires several hundred hours. If the input data required can be reduced by a significant margin, then the barriers to entry will be lowered substantially.
Generative adversarial networks , on the other hand, are aimed at auto-generating labeled data, such as images and sounds, which can be used for deep learning. Hou pointed out, for example, that when applied to facial recognition, GAN can produce an image of the human face that satisfies recognition conditions. GAN is comprised of two neural networks: the first is a generative network, which can use inputted data to generate labeled data; the other is a recognition network, which is able to distinguish if the samples produced by the generator meet certain conditions. Hou likened the way the two function to a forger and an art critic. The models thrown up by the generative network are like the forger, seeking to hoodwink the art critic into thinking the artwork it has generated is real. In the adversarial process between the two AI models, they continue to improve their skills, until they can work without any human guidance at all. GAN can produce all kinds of data, providing a range of applications, like time series prediction, image inpainting, 2D to 3D transformation, encryption, and labeling unlabeled data. It can also create artworks and create music.
When AI is trained without sufficient data, the AI may internalize biases in its input data. One example of this was the Tay AI released by Microsoft, which posted hateful tweets on Twitter and which, as s result, had to be turned off after just one day. Any prejudice in the data fed into the AI will be reflected in its algorithms. There have also been studies suggesting that courts in the United States that use AI to judge whether prisoners should be granted bail have shown racial biases, for example.
Another challenge facing AI is that traditional deep learning algorithms find it hard to accumulate skills or knowledge, in that normally each separate application requires a new model. Expertise at the game Go, for example, will not give an AI any expertise at chess or Chinese chess, for example, as it isn’t able to transfer what it has learned to different applications. Google Deepmind, the creators of AlphaGo now claim to have developed AlphaZero, a single algorithm capable of besting humans in chess, go and shogi within 24 hours, suggesting that things are changing in this respect. Hou pointed to the example of voice recognition, in that samples must be collected separately for each individual language to form separate algorithms, as opposed to AI being able to learn a language more quickly based on the information on semantic structure it has already learned in a different language.
In an effort to resolve this issue, there have been attempts made to graph languages into semantic units, which could result in cross-language training. The common features of languages could be harnessed to allow the accumulation and transfer of study, which looks at common features, making use of different knowledge at different levels.
Hou stated that there has been a shift away from the traditional x86 architecture CPUs, which are not the most appropriate for deep learning calculations. A range of new chips which attempt to mimic mammalian brains to some extent have been released in an attempt to facilitate the development of AI.
Mammalian Brains |
Computers |
Parallel distributed architecture |
Serial architecture |
Low power (25W), small footprint (1 liter) |
High power (100MW), large footprint (40M liters) |
Asynchronous (no global clock) |
Synchronous (global clock) |
Analog computing; Digital communication |
Digital computing and communication |
Integrated memory and computation |
Memory and computation are clearly separated |
Intelligence via learning through BBE interactions |
Intelligence via programmed algorithms/rules |
Composed of noisy components and operates at low speeds (< 10 Hz) |
Precisions in components and operates at very high speeds (GHz) |
Spontaneously active |
No activity unless instructed |
Source: ‘Rebooting The IT Revolution: A Call to Action’; SIA/SRC; Sept. 2015
Hou listed some of the most renowned AI chips on the market, including Google’s Tensor Processing Unit (TPU), IBM’s TRUENORTH brain-inspired processor, Intel’s Xeon Phi CPU/FGPA/NPU, Qualcomm’s Zeroth Processor NPU and the NVIDIA P100 GPU.
Hou stated that one of the big challenges for AI will be the migration of computing from the cloud to the edge, as many computations will need to be made in shorter periods than the cloud can facilitate. Hou suggested that quantum computing will be the next big trend in the tech world over coming years, with the launch of IBM Q and other companies, including Google Microsoft and Alibaba all developing quantum computers. The quicker-than-expected development of quantum computers could mean that quantum computing may replace large cloud data centers in the market for AI operations.
Another issue that AI has yet to overcome is the fusing of different sensor functions, to create different types of data which can be used simultaneously, in the same way that when humans are listening to another person speak, they can sometimes make inferences as to what they are saying from the shape of their mouths even if there is a lot of background noise. Google is currently working with MIT to find solutions to this problem.
Hou stated that there is likely to be a larger shift away from the current model of supervised learning, towards unsupervised learning and reinforcement learning.
At the conference the College of Electrical Engineering and Computer Science of National Taiwan University, which was also hosting the conference, gave a rundown of their patent holdings across different fields and in which countries they hold patent rights (See charts below).
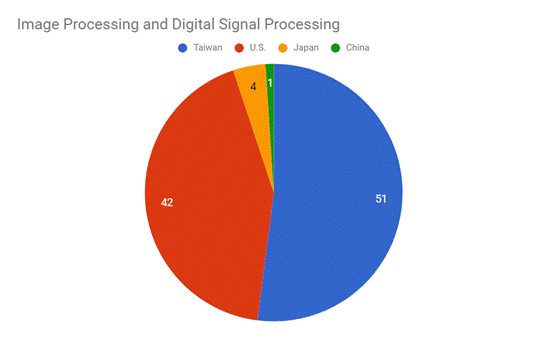 Source: AI Smart Lives Patent Trends and Strategies; Charts compiled by Conor Stuart
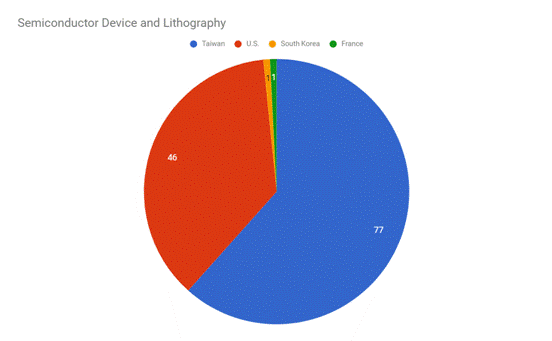 Source: AI Smart Lives Patent Trends and Strategies; Charts compiled by Conor Stuart
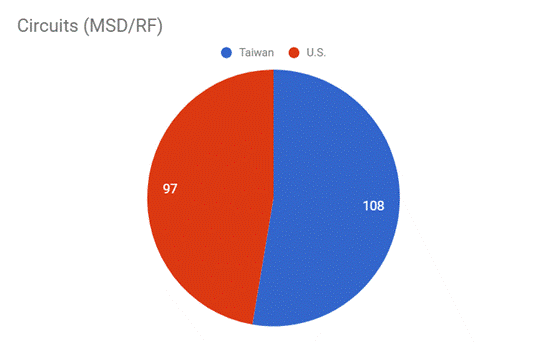 Source: AI Smart Lives Patent Trends and Strategies; Charts compiled by Conor Stuart
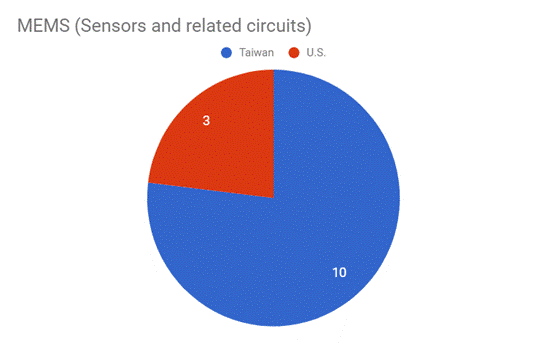 Source: AI Smart Lives Patent Trends and Strategies; Charts compiled by Conor Stuart
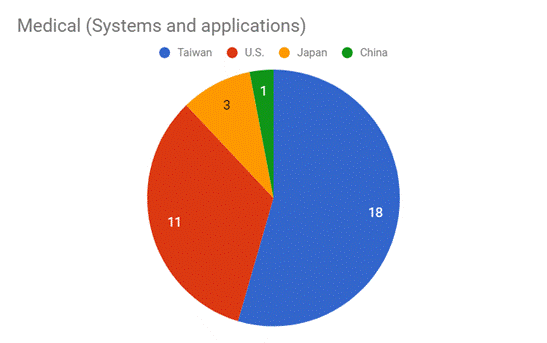 Source: AI Smart Lives Patent Trends and Strategies; Charts compiled by Conor Stuart
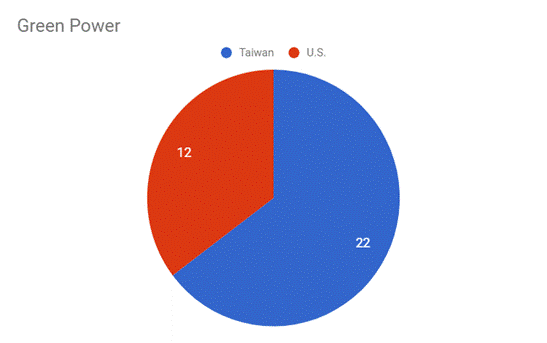 Source: AI Smart Lives Patent Trends and Strategies; Charts compiled by Conor Stuart
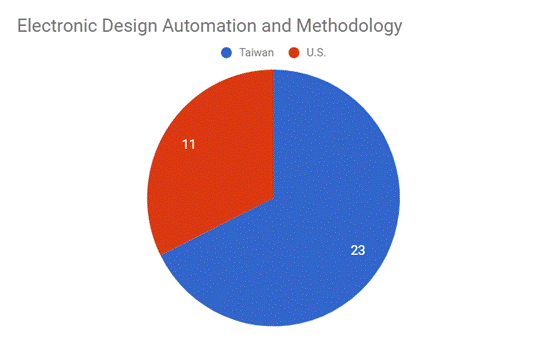 Source: AI Smart Lives Patent Trends and Strategies; Charts compiled by Conor Stuart
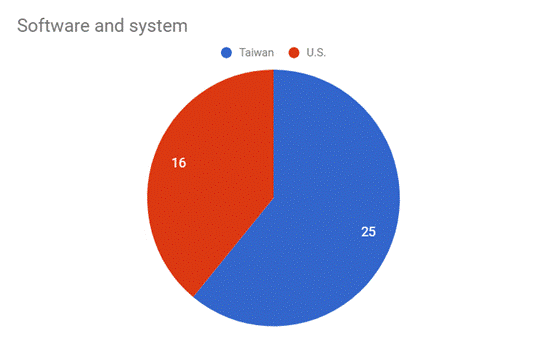 Source: AI Smart Lives Patent Trends and Strategies; Charts compiled by Conor Stuart
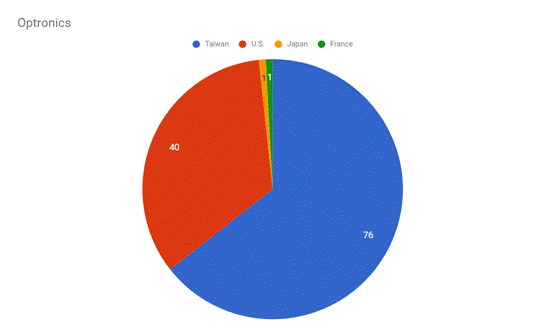 Source: AI Smart Lives Patent Trends and Strategies; Charts compiled by Conor Stuart
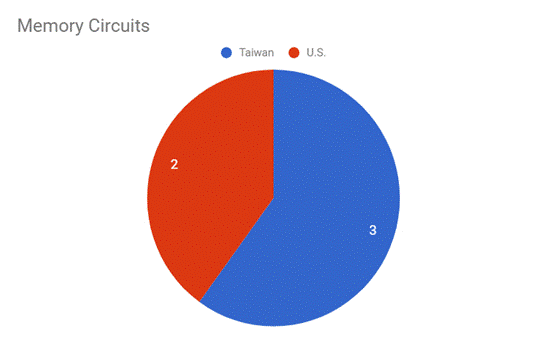 Source: AI Smart Lives Patent Trends and Strategies; Charts compiled by Conor Stuart
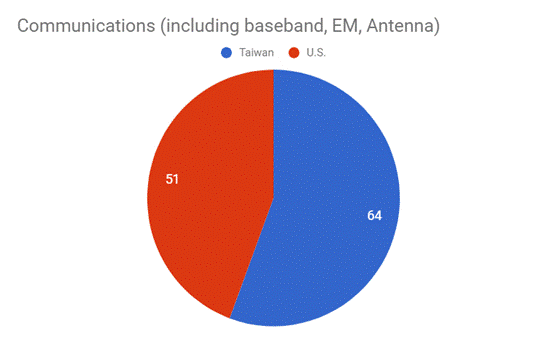 Source: AI Smart Lives Patent Trends and Strategies; Charts compiled by Conor Stuart
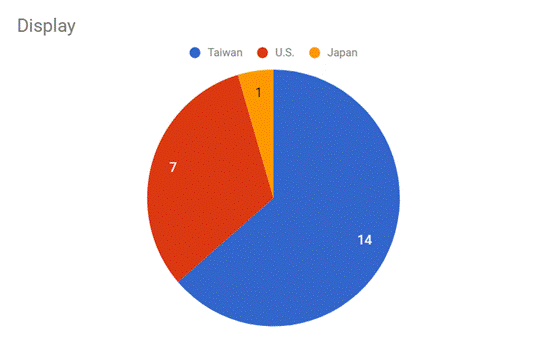 Source: AI Smart Lives Patent Trends and Strategies; Charts compiled by Conor Stuart
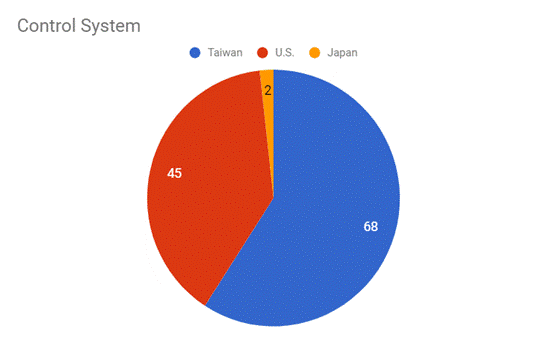 Source: AI Smart Lives Patent Trends and Strategies; Charts compiled by Conor Stuart
Source of Main Article Image: MaxPixel
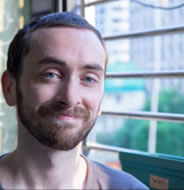 |
|
Author: |
Conor Stuart |
Current Post: |
Senior Editor, IP Observer |
Education: |
MA Taiwanese Literature, National Taiwan University
BA Chinese and Spanish, Leeds University, UK |
Experience: |
Translator/Editor, Want China Times
Editor, Erenlai Magazine |
|
|
|
Facebook |
|
Follow the IP Observer on our FB Page |
|
|
|
|
|
|